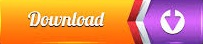
In addition, we use the hidden variable region r to explore the interaction between time preference and geographical preference. TARE consists of two parts: embedding of events and prediction of event occurrence, which will be described below. Figure 2 shows the graphical representation of TARE.
Poi organizer full#
Table 2 lists the abbreviation and full name of different concepts in this paper. Table 1 lists the notations of our model. To this end, TARE uses two hidden variables ( r, z) to discover the relationship between time, POI category and geographic information.
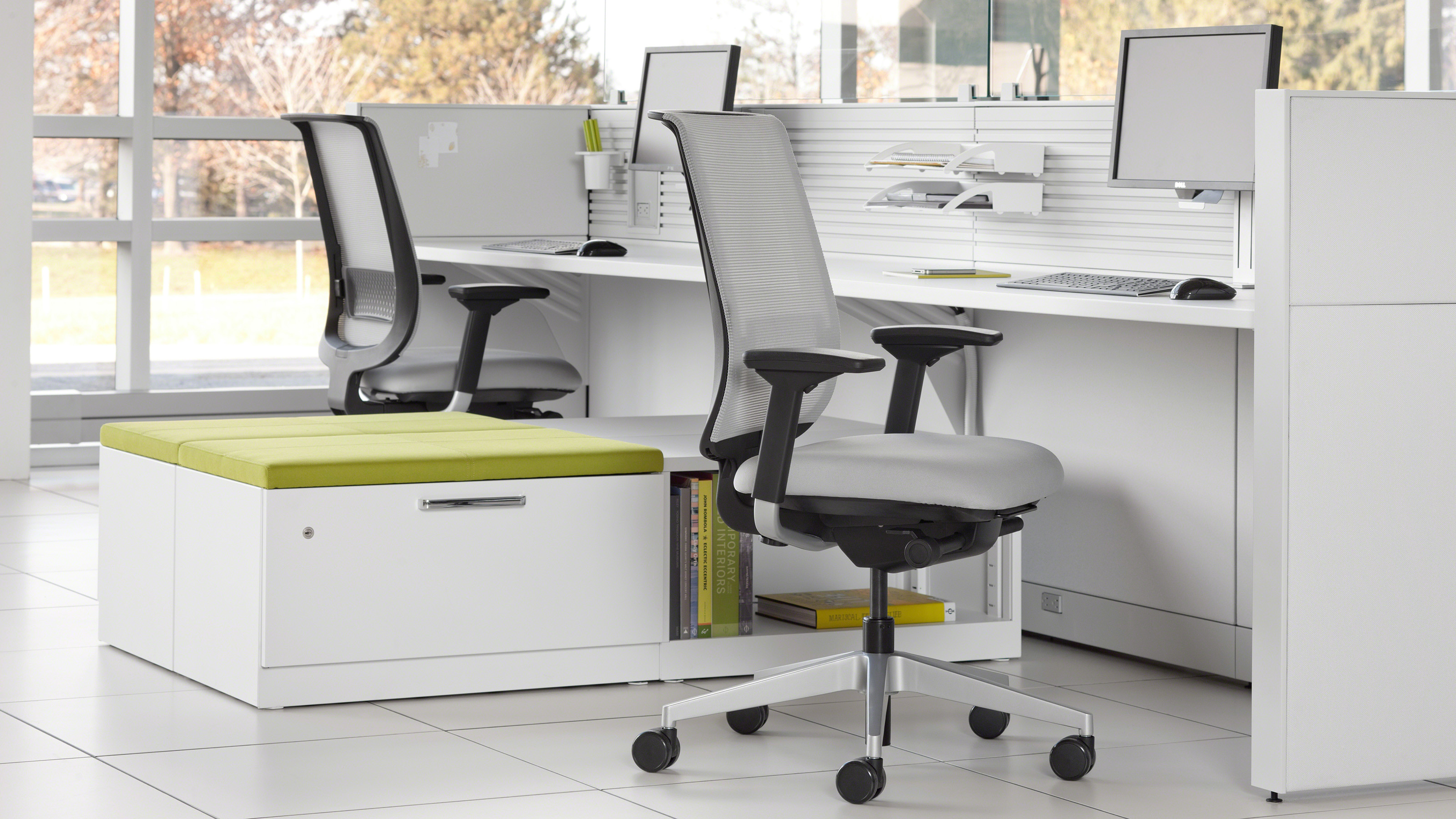
In other words, time factors affect user behavior all the time. User behavior has a similar periodic pattern, and this is manifested in the fact that users always visit similar POIs at specific times . TARE divides the factors that influence user behavior into four categories: geographic information, time factor, POI category, and personal preference. So, the embedding methods should take into account the time and geographic location for better recommendation performance.Īccording to the above process, this paper proposes a new embedding method called Topic And Region Embedding (TARE), which embeds events according to user preferences, event time, event location and POI by simulating the user decision process. Event locations can filter out POIs that are too far away and thus improve the accuracy of user behavior predictions. The certain time and user history check-in records can be used to infer the user preferences at event time. Second, we notice that the effectiveness of personalized recommendation is closely related to the current time and location. For example, users may like to visit a POI near their home at night while preferring to visit a POI near their work location during the day (time affects the user’s geographic preferences). Notice that the intrinsic relationship between different factors has a great impact on the user’s behavior. Firstly, the existing embedding methods consider multiple factors by embedding a separate model for each factor without exploring the intrinsic relationship between different factors. Nevertheless, these existing embedding methods have certain deficiencies.

Extensive experiments on three location-based social network datasets show that TARE achieves better performance in recommendation accuracy than existing state-of-the-art methods. The results of TARE not only take various factors and their interaction into consideration but also consider the time and geographic location of events. In this paper, we propose a probabilistic embedding model called Topic And Region Embedding (TARE), which embeds events by simulating the users’ decision-making process. In other words, it is necessary to predict the most likely visiting event, including users, POI, event time, and event location. Therefore, it is necessary to recommend the right POI according to the time and geographic location of the user. It is obvious that users would check into a POI which fits their interests, even if the current location is far away from the POI or the time is inappropriate. In addition, we notice that the effectiveness of personalized recommendation is closely related to the current time and location. As a result, the interaction amongst various factors cannot be captured properly. The existing embedding techniques deal with multiple factors by embedding a separate model for each factor. Location-based social networks (LBSNs) have collected massive geo-tagged information, enabling the derivation of user preference for point of interests (POIs) in support of personalized recommendation.
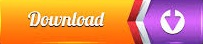